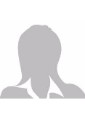
Neeru Rathee
Guru Gobind Singh Indraprastha University, India
Title: Detecting facial action units for analyzing facial expressions caused due to pain
Biography
Biography: Neeru Rathee
Abstract
Pain assessment is the key concern of medical practioners since last many years. The commonly adopted non-invasive methods include visual analog scale, clinical interviews, self-report by patients and observer rating which can be used conveniently without applying any advance technology or special skill. But, these methods are highly subjective and cannot be applied for cases where monitoring is required for lengthy period of time. One solution for pain assessment is by analyzing facial expressions. The atomic unit of facial expressions is facial action unit (AU), which in combination with each other are responsible for any facial expression. The change in facial expressions caused due to pain has been successfully coded by Prkachin by introducing the action units responsible for pain. According to Prkachin, pain can be represented by AUs with numbers 4, 6, 7, 9, 10, 12, 25 and 43. So, intensity of these AUs was computed for pain detection. The information about facial expressions representing these AUs is retrieved by two different features: geometric features and texture features. The geometric features are extracted by modeling the facial features and then by computing the geometric distances and ratios between various landmark points representing facial features. Texture features are computed by pixel based methods and include Gabor features. These two features are combined by simply concatenating them and then fed to support vector machine, which is one of the well-established tool of machine learning, for AU intensity estimation. The approach was evaluated on UNBC-McMaster database and resulted in 95% accuracy for pain detection.